This article shares analysis in solution cycle for the Open Exchange application TOOT ( Open Exchange application )
The hypothesis
A button on a web page can capture the users voice. IRIS integration could manipulate the recordings to extract semantic meaning that IRIS vector search can then offer for new types of AI solution opportunity.
.png)
The fun semantic meaning chosen was for musical vector search, to build new skills and knowledge along the way.
Looking for simple patterns
The human voice talking, whistling, humming has constraints realized in many historical low bandwidth data encodings and recording media.
How little information is needed to distinguish one musical sound from another as it relates to musical voice input?
Consider the sequence of musical letters:
A, B, C, E, A
Like in programming with ASCII values of the characters:
* B is one unit higher than A
* C is one unit higher than B
* E is two unit higher than C
* A is four units lower than E
Represented as a numerical progression between two numbers:
+1, +1, +2, -4
One less information point is needed than the original count of notes.
Logically testing after the first coffee of the morning the humming became:
B, C, D, F, B
Note that the numerical sequence still matches for this elevated pitch as well.
This demonstrates that a numeric progression of difference in pitch seems more flexible to match user input than actual notes.
Note Duration
Whistling capability has a lower resolution than represented by traditional musical manuscripts.
A decision was made to resolve only TWO musical note duration types:
- A long note that is 0.7 seconds or longer
- A short note that is any less than 0.7 seconds.
Note Gaps
The gaps have quite poor resolution so we use only one information point.
A decision was made to define a note gap as:
a pause between two distinct notes of more than half a second.
Note Change Range
A decision was made to limit the maximum recordable transition between one note and another of 45 notes pitch.
Any note change in user input that exceeds this limit is truncated to either +45 or -45, depending on whether the pitch was increasing or decreasing. Unlike actual notes there is no penalty on the usefulness of a continuing tune search sequence.
+1 0 +2 -4 +2 +1 -3
Can be trained to be semantically close to following change sequence in red.
+1 1 +2 -4 +2 +1 -3
Single channel Input
A voice or whistle is a simple instrument with only one note at a time.
However this needs to be match against music ordinally composed of:
* Multiple instruments simultaneously
* Instruments that play chords ( several notes at a time )
Generally a whistle tends to follow ONE specific voice or instrument to represent a reference music.
In physical recordings scenarios individual voices and instruments can be recorded in distinct tracks which are later "mixed" together.
Correspondingly encoding/decoding utilities and data formats can also preserve and utilize "tracks" per voice / instrument.
It follows that hum / whistle input should search against the impression of each individual voice and instrument track.
Several "musical" encoding models / language formats were considered.
The simplest and mature option was utilizing MIDI format processing to satisfy determining reference encodings for whistle match and search.
Vocabulary summary
Apart from the usual begin, end and pad token sequences information points
- 45 Long notes decreasing each with a specific magnitude of change
- 45 Short notes decreasing each with a specific magnitude of change
- Repeat same note with short duration
- Repeat same note with long duration
- 45 Long notes ascending each with a specific magnitude of change
- 45 Short notes ascending each with a specific magnitude of change
- A gap between notes
Synthetic data
IRIS globals are very fast and efficient at identifying combinations and their frequency across a large dataset.
The starting point for synthetic data was valid sequences.
These were modified cumulatively in different ways and scored by deviation:
- SplitLongNote - One long note becomes two short where the second becomes a repeat
- JoinLongNote - Two short notes where the second is a repeat, becomes a single long note.
- VaryOneNote ( +2, +1, -1 or -2 )
- DropSpace - Remove gap between note
- AddSpace - Add gap between note
Next these scores are effecively layered in a global for each result.
It means that where another area of note-change-sequence in a track stream is closer to a mutated value, the highest score was always picked.
Dev Workflows
Search Workflow
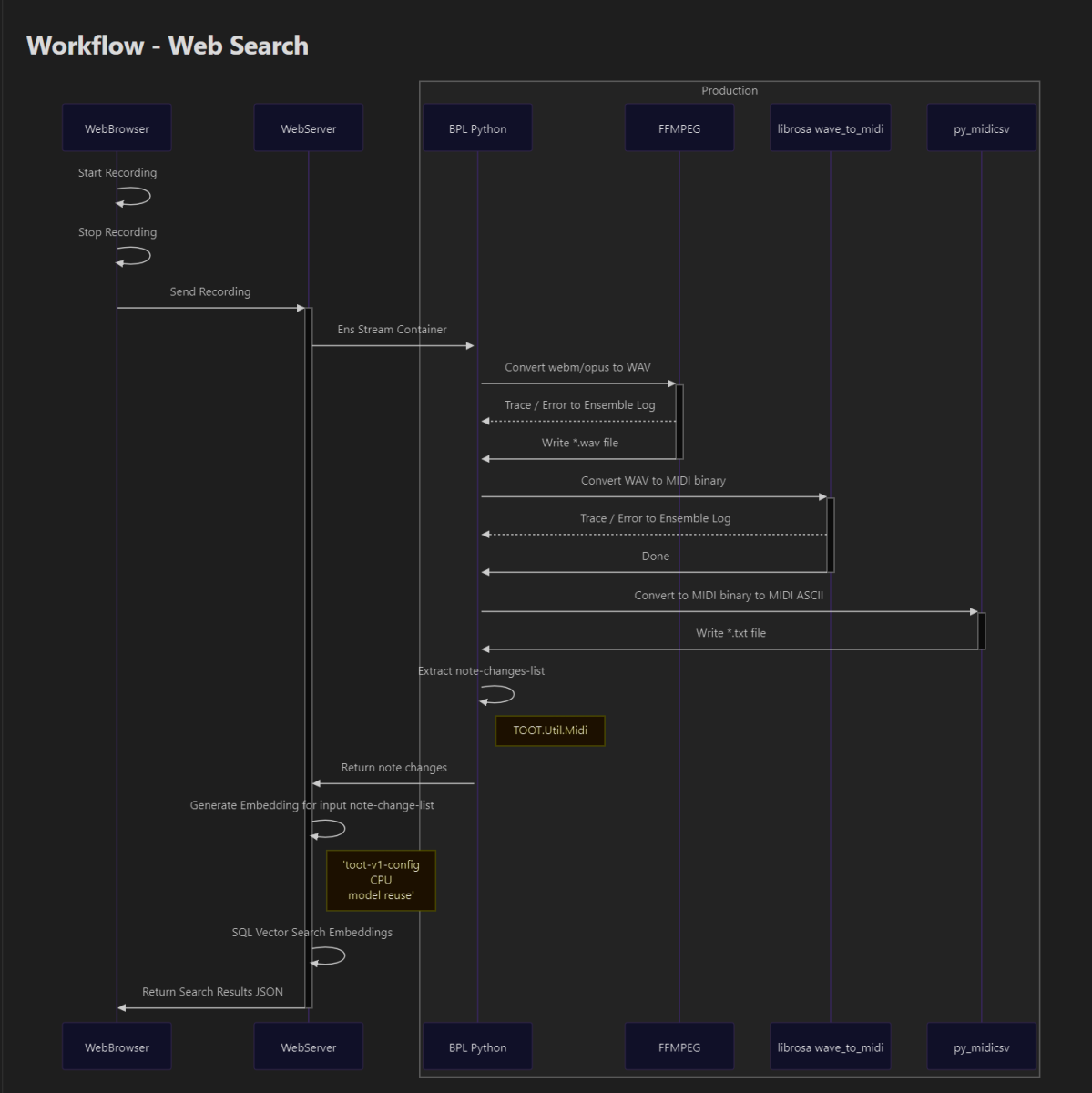
DataLoad Workflow
Vector Embeddings were generated for multiple instrument tracks (22935 records), for sample tunes (6762 records)
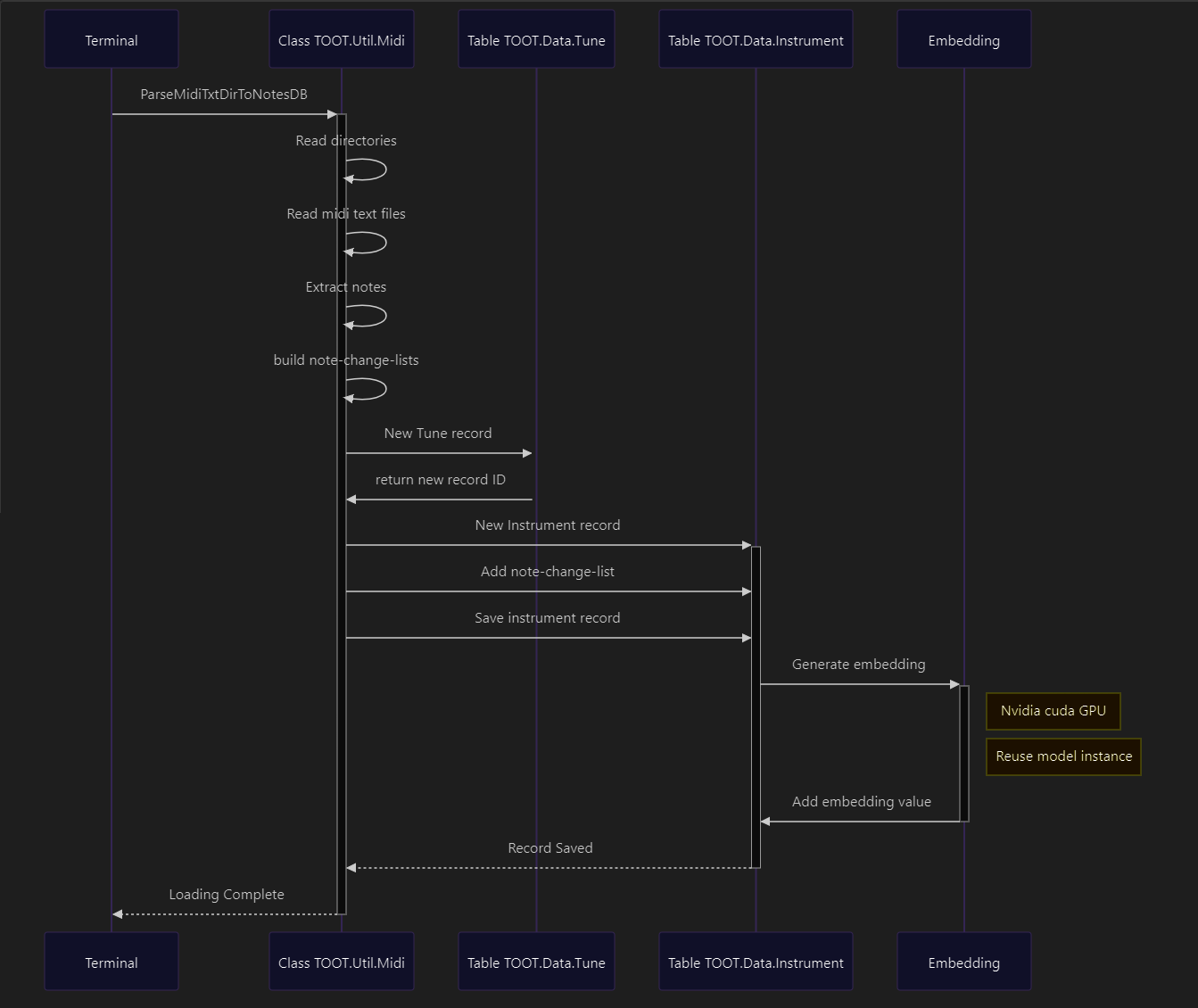
Training Workflow
Two training experiments:
Unsupervised - Max 110,000 records ( 7 hours processing )
Similarity score supervised - Maximum 960,000 records (1.3 days processing)

Further requirements to explore
Better similarity scoring
The current implementation iteration is pruning by scores too hard.
Review cut-off for including low occurance and high occurance sequences in dataset.
Filtering background noise
In midi format the volume of voices or instruments are important in terms of the actual user and background noise. Possibly this gives some opportunity to clean / filter a datasource. Maybe exclude tracks that would never be referenced by human input. Currently the solution excludes "drums" by instrument track by title and by analysis of some progression repetition.
Synthetic midi instruments
The current approach of matching voice to instruments was to trying and side-step a mismatch in instrument characteristics, to see if that was good enough.
A canditate experiment would be add some characteristics back from user input while at the same time pivoting synthetic training data to have more human characteristics.
MIDI encodes additional information with pitch bending to achieve a smoother progression between notes.
Would be an avenue to explore to extending and refining the way WAV to MIDI translation is done.
Finally
Hope this was interesting diversion from the day-today and that you enjoy the app or that it inspires some new ideas..