Thanks Robert. But if I do the lookup tables manually in major sites go-lives, I'd simply use Excel and Notepad++. It's not just quicker and simpler; also most real sites under RFC control would not allow you do such dozens of DSNs in Live environments, even for a hot fix in go-live deadlines. BTW, I love to work with engineers too, and LLMs and AIs. I never saw any engineer in deliberate competition with GPTs yet, so far.
And please see the Disclaimer section at its end. It's not the purpose of this post.
You are absolute right, Tete. It's just a boilerplate structure. And apparently it does JavaScript and Python better than COS for now. Due to the nature of current ChatGPT, every time it would compose slightly different answers & samples for you, so unfortunately I can't replicate the answer - otherwise I'd love to feed your exact comments back into it, to see whether its Reinforcement Learning style part is able to follow up and really "learn", and how much it could revise its sample step by step to an accurately working & testable sample. I assume there seems no reason it can't pick it up over the time.
For example, if I ask the same question again, it would answer slightly differently
ChatGPT:
Sure, here's an example of how to transform an HL7 V2 message to a FHIR STU3 Patient resource using the Data Transformation Language (DTL) in InterSystems Caché:
- First, create a new DTL class in InterSystems Caché.
- In the DTL class, define two message classes: one for the input HL7 V2 message and one for the output FHIR STU3 resource. Here's an example of what the message classes might look like:
Class HL7v2Message Extends %SerialObject
{
Property MSH As %String(MAXLEN = 100, TRUNCATE = 1, XMLPROJECTION = "attribute") [ Required ];
Property PID As %String(MAXLEN = 500, TRUNCATE = 1, XMLPROJECTION = "element") [ Required ];
}
Class FHIRPatient Extends %SerialObject
{
Property resourceType As %String(MAXLEN = 100, TRUNCATE = 1, XMLPROJECTION = "attribute") [ InitialExpression = "Patient" ];
Property id As %String(MAXLEN = 100, TRUNCATE = 1, XMLPROJECTION = "attribute");
Property active As %Boolean(XMLPROJECTION = "element");
Property name As list Of FHIRHumanName(XMLPROJECTION = "element");
Property telecom As list Of FHIRContactPoint(XMLPROJECTION = "element");
Property gender As %String(MAXLEN = 10, TRUNCATE = 1, XMLPROJECTION = "element");
Property birthDate As %String(XMLPROJECTION = "element");
}
- Define a new DTL transformation method in the class that takes an input HL7v2Message object and returns a FHIRPatient object. Here's an example of what the DTL method might look like:
Method HL7v2ToFHIRPatient(input As HL7v2Message) As FHIRPatient [ CodeMode = objectgenerator, Internal ]
{
Set output = ##class(FHIRPatient).%New()
// Parse MSH segment
Set output.id = input.MSH.%split("^",1)
// Parse PID segment
Set output.active = 1 // patient is always active in HL7 v2
Set output.name = ..ParseName(input.PID.%split("^",5))
Set output.telecom = ..ParseTelecom(input.PID.%split("^",13))
Set output.gender = input.PID.%split("^",8)
Set output.birthDate = ..ParseDate(input.PID.%split("^",7))
Quit output
}
Method ParseName(name As %String) As list Of FHIRHumanName
{
Set names = ##class(%List).%New()
Set nameObj = ##class(FHIRHumanName).%New()
Set nameObj.family = name.%split("^",1)
Set nameObj.given = name.%split("^",2)
Do names.Insert(nameObj)
Quit names
}
Method ParseTelecom(telecom As %String) As list Of FHIRContactPoint
{
Set contacts = ##class(%List).%New()
Set contactObj = ##class(FHIRContactPoint).%New()
Set contactObj.system = "phone"
Set contactObj.value = telecom.%split("^",1)
Do contacts.Insert(contactObj)
Quit contacts
}
Method ParseDate(date As %String) As %String
{
Set year = $extract(date, 1, 4)
Set month = $extract(date, 5, 6)
Set day = $extract(date, 7, 8 ... ...
... ...
Here we seem to see, it doesn't learn much about the internal class library yet - it seems trying to construct FHIR etc classes from the ground up without knowing the existing FHIR plumbing yet. Not sure how it passed the Google L3 Engineer test, but it would fail the HealthConnect certification for now - it has a long way to learn, indeed, possibly also depending on whether we really want it to learn too quick? :)
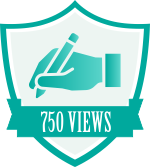
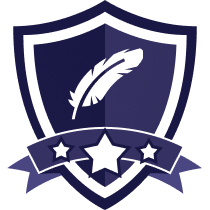
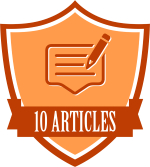
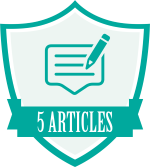
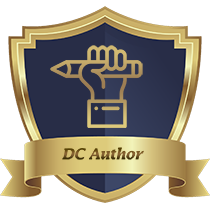
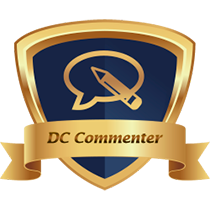
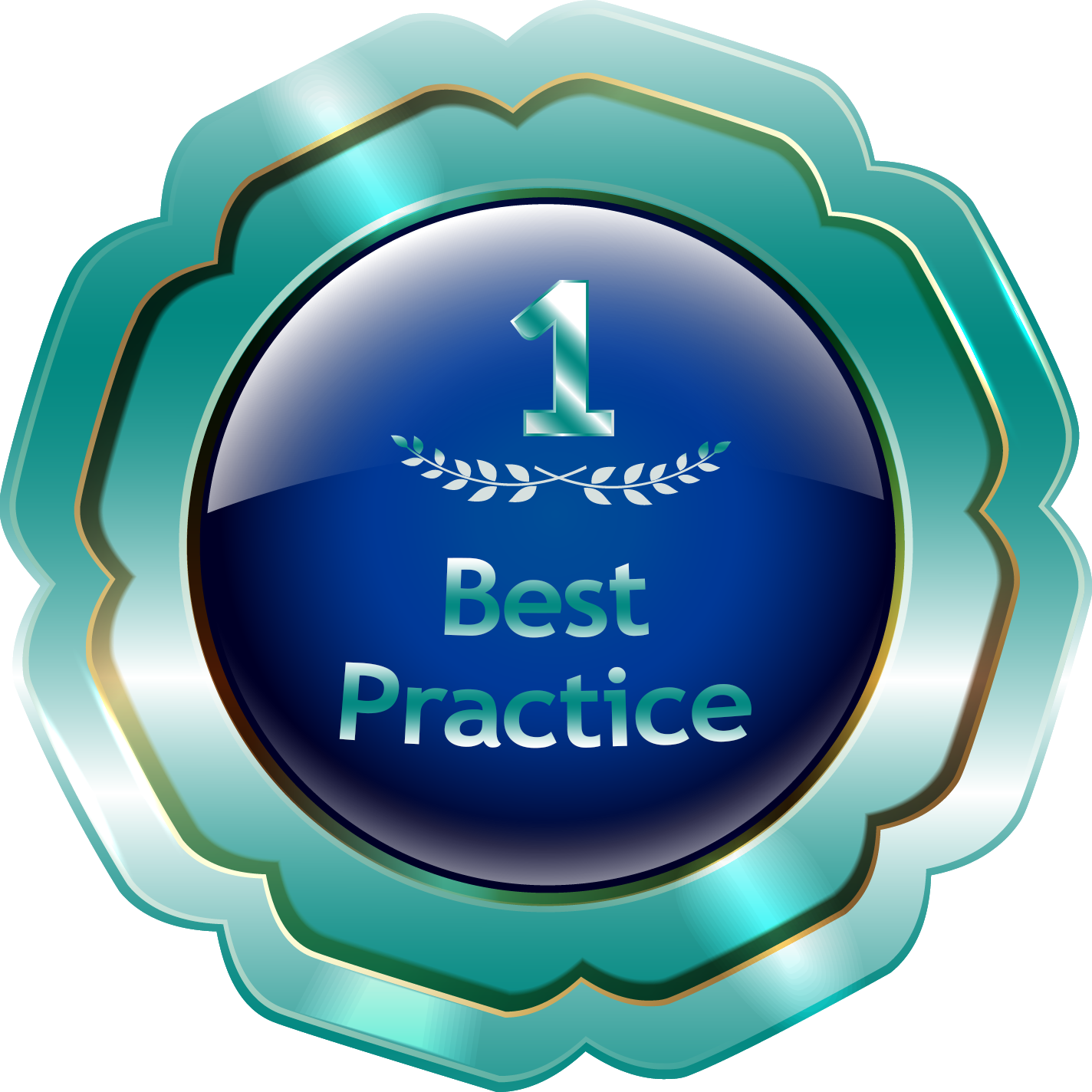
https://www.bbc.co.uk/news/technology-65110030
Agreed with the risks, so I appended a line of excuse in Disclaimer section.
Not sure we should stop or slow down the phenomenal progress of AGIs, but I do agree sovereign states should speed up legislations. Also the theoretical researches on its mathematical nature. :)